Numenta hosted a Brains@Bay meetup on December 15, 2021, entitled Sensorimotor Learning in AI. Launched in 2019, Brains@Bay MeetUps cover topics relevant to both neuroscience and the artificial intelligence and machine learning landscape. We’ve been fortunate to have some of the top experts in AI and ML as guest speakers over the years, and the sensorimotor MeetUp followed in that tradition. It featured Richard Sutton, renowned as the father of reinforcement learning and one of the most influential figures in the field of AI.
The term sensorimotor learning conveys how the brain learns by creating a model of the world through sensory observation of our environment as we move. When we observe and interact with our environment, we discover the structure of things. Numenta has been doing neuroscience research on sensorimotor learning for more than fifteen years and we’ve published several papers on it. We have a deep understanding of sensorimotor learning in the brain, and we were eager to hear from a panel of researchers who study sensorimotor learning through the lens of AI.
The speakers for this MeetUp were: Richard Sutton of DeepMind and the University of Alberta; Clément Moulin-Frier of Flowers Laboratory; and Viviane Clay, of Numenta and the University of Osnabrück. Each speaker highlighted new concepts of how machine learning systems can achieve greater flexibility, robustness, and intelligence by incorporating sensorimotor learning. The speakers joined in a panel session, covering the general field and answering questions from the audience. Their talks warrant a recap below and we also recommend a deep dive by checking out their full presentations.
Experiential or Objective
Numenta co-founder Jeff Hawkins set the tone for the MeetUp by introducing Richard as a distinguished researcher whose work in reinforcement learning shows that sensorimotor systems are “a prominent feature of AI.” Richard’s presentation, The Increasing Role of Sensorimotor Learning in AI, highlighted sensorimotor experience—the sensations and actions that define interactions—as conceptually bridging machine learning systems and biological systems. He posed a key question in AI: “will intelligence ultimately be explained in experiential terms or objective terms?”
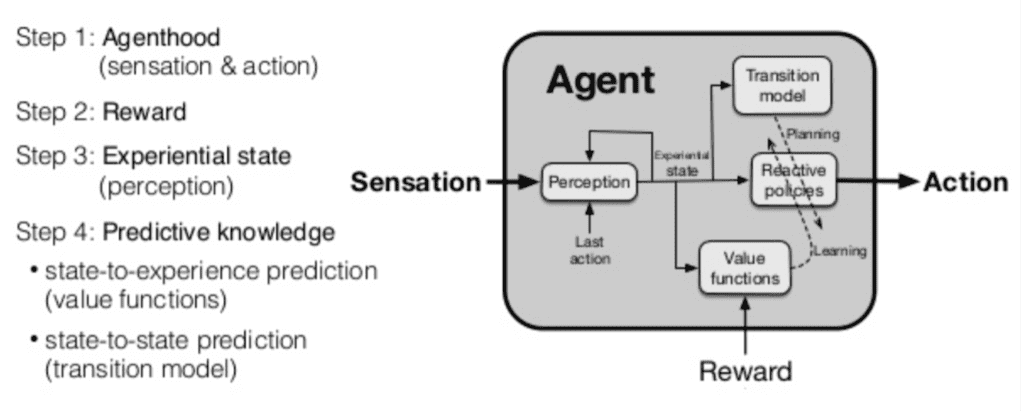
Richard emphasized that predictive knowledge has traditionally not been considered in experiential terms, but that is changing. “Classically, world knowledge has always been expressed in terms far from experience, and this has limited its ability to be learned and maintained,” he said. “Today we are seeing more calls for knowledge to be predictive and grounded in experience. After reviewing the history and prospects of the four steps, I propose a minimal architecture for an intelligent agent that is entirely grounded in experience,” he concluded.
Skill Acquisition
Clément Moulin-Frier’s presentation was entitled, Open-ended Skill Acquisition in Humans and Machines: An Evolutionary and Developmental Perspective. Clément proposed “a conceptual framework sketching a path toward open-ended skill acquisition through the coupling of environmental, morphological, sensorimotor, cognitive, developmental, social, cultural, and evolutionary mechanisms.“
Clément illustrated parts of this framework through computational experiments. He highlighted research that showed the key role of intrinsically motivated exploration in behavioral regularity and diversity. The experiments demonstrated two main points:
- Some forms of language can self-organize out of generic exploration mechanisms without any functional pressure to communicate.
- Language can be recruited as a cognitive tool that enables compositional imagination and bootstraps open-ended cultural innovation.
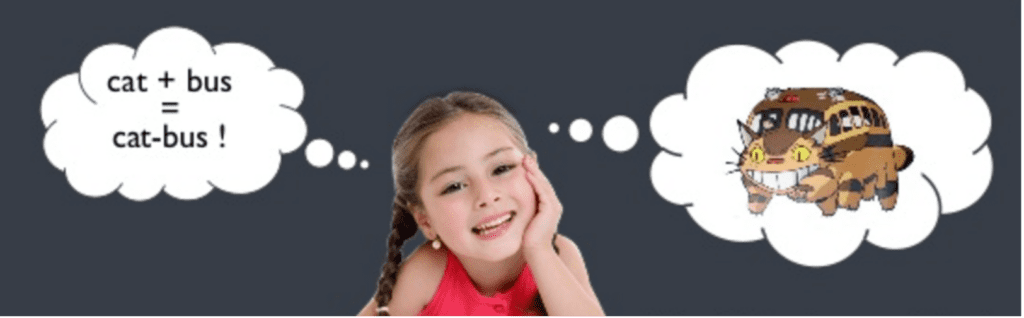
Learned Representations
Numenta’s Viviane Clay then began her presentation, The Effect of Sensorimotor Learning on the Learned Representations in Deep Neural Networks. Citing original research, she emphasized that the representations learned through very weakly supervised or self-supervised exploration are more structured and meaningful than strongly supervised learning. “They encode action-oriented information in very sparse activation patterns,” she said. “The encodings learned through interaction differ significantly from the encodings learned without interaction.”
Viviane highlighted data that showed the capabilities for DNNs to learn similarly to the brain, through sensorimotor interaction with the world. Much of the presentation focused on a “fast concept mapping” paradigm that was aligned with Jeff Hawkins’ Thousand Brains Theory. The presentation emphasized the utility of sparse representations learned through self-supervised, sensorimotor interaction with the world.
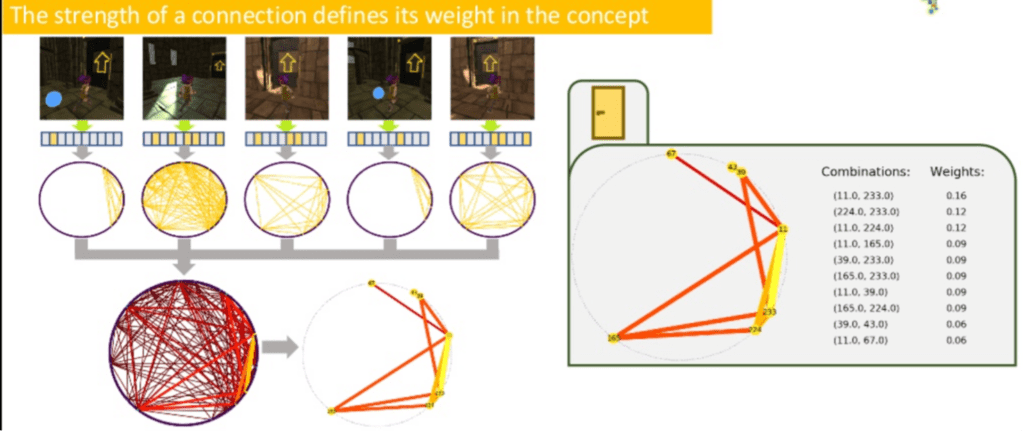
MeetUp Management
The talks described above touch on many such subjects, including facets of sparsity, objective versus experiential learning, the role of language, implementation of rewards, and much more. While sensorimotor learning is a key facet of AI, there are many other topics we would like to tackle in future MeetUps.
Brains@Bay is organized to address the overarching question, “what new lessons from the brain can help us move beyond the limitations of machine learning?” The MeetUp is regularly held and expertly managed by Numenta’s Charmaine Lai, who accepts topic suggestions at clai@numenta.com. If you have comments, questions, or ideas for future talks, please reach out.
Sensorimotor Citations
As a final note for his talk, Clément wished to acknowledge:
- Eleni Nisioti, Katia Jodogne-del Litto, Clément Moulin-Frier. Grounding an Ecological Theory of Artificial Intelligence in Human Evolution, EcoRL workshop @ Neurips 2021
- Colas, Karch, Lair, Dussoux, Moulin-Frier, Dominey & Oudeyer. Language as a cognitive tool to imagine goals in curiosity-driven exploration. NeurIPS 2020
- Moulin-Frier, Nguyen and Oudeyer (2014) Self-organization of early vocal development in infants and machines: the role of intrinsic motivation. Frontiers in Psychology
Viviane acknowledged:
- Viviane Clay, Peter König, Kai-Uwe Kühnberger, Gordon Pipa.Learning sparse and meaningful representations through embodiment. Neural Networks 134: 23-41 (2021)
- Viviane Clay, Peter König, Gordon Pipa, Kai-Uwe Kühnberger.Fast Concept Mapping: The Emergence of Human Abilities in Artificial Neural Networks when Learning Embodied and Self-Supervised.